Traditionally, IT admins use MDM to provision, manage, and secure laptops and corporate phones to employees — bring your own device (BYOD) or corporate-owned, personally-enabled (COPE) — with the goal of preventing employees from unknowingly (or maliciously) enabling a corporate data breach. In other words, to stop bad actors from accessing sensitive data while allowing as much productivity as possible.
In this world, devices are tied to users, and users are the first-class citizens from an IT perspective. When the user logs in, they get their settings and apps, and generally have access to whatever they want within those parameters. The whole policy is centered around the user’s interaction with their device. If the employee leaves the company or their role changes and they need new apps or access to other new things, the IT admin changes or wipes the user’s profile. When the user refreshes and logs back in, the device gets updated settings. Again, it’s all down to the user.
While this traditional device management scenario certainly still exists, there’s an entirely new class of device use cases that are wildly different and introduce completely different compliance and security challenges — use cases where the device itself must be treated as a first-class citizen. These are the ones I want to talk about. (They’re also far more interesting, challenging, and emergent.)
Ultimately, the question is: What do compliance and security look like when you deploy devices in decentralized environments, often in different conditions, and without one specific user?
For example, I have seen devices in the hands of operators who should not have full access to the device. The barista at your coffee shop does not need access to the POS terminal’s device settings. A traveler in an airport should not be able to download new apps onto the self-check-in kiosk. The traditional MDM assumption is that if a human holds a device, they should have full access. You can no longer make that assumption. We can’t assume that the surrounding ecosystem is secure.
You must be better at noncompliance risk detection to elevate your security posture. In many cases, it is too late to say, “Oh no! This device is noncompliant!” You must know the risk before it is a problem and then take proactive steps to mitigate it. One of the more straightforward tools is drift monitoring. If a device has access to an app or content that is not part of its desired state, it is in drift. Send a drift alert and remotely remediate it by removing the app and locking the device down.
The more advanced tools for proactive mitigation are great use cases for AI/ML, such as identifying security issues inside apps.
Right now, if you deploy apps through the Google Play Store, you rely on Google, or if you use the App Store, you rely on Apple to identify app issues and make sure they’re safe. But there could be more mischief (or unintentional vulnerabilities) hidden inside. An example is using AI/ML to provide greater confidence with positives and negatives.
What I mean by that is that if I say I am 100% confident that there’s no risk of app security vulnerability, I want it to definitely be 100%. If my system says 90%, it may be 100%, or it may be 90%. There's still a lot of value in making sure that at least 100 means 100. Essentially, having a false negative is way better than a false positive. We can use ML to tune systems to have more false negatives and not more false positives so you’re not lulled into a false sense of confidence.
This is actually part of a broader noncompliance bucket with anomaly detection. Because newer MDM solutions give a high degree of granularity in telemetry data, all their data needs to — if consumed well and if structured well enough — provide immense power for operators to identify noncompliance across their fleet. As fleets grow, identifying risk is like finding a needle in a haystack. Enter anomaly detection. If you have 1000s of devices, how do you know if one of them is undergoing a security breach at any given moment? You can use and analyze patterns, such as traffic patterns, to see if a device is getting pings from a new IP that they’re not supposed to or at a much higher frequency than expected.
When you can’t rely on users and user profiles for security protection and mitigation like the traditional security use case for MDM, the next generation of MDM must leverage alternate methods to get and stay ahead of device risk. As we’ve covered, using AI/ML for risk tuning and anomaly detection are two critical tools. This becomes much easier once the ML workflow is integrated with the MDM provider because it leverages the underlying telemetry and management backbone of the provider to consume the data and turn that into insights for users.
As always, there’s much more to unpack in this space, but I hope this starts new thought processes and conversations about the coming requirements for MDM, as well as for its users and buyers.
FAQ
Keep Exploring
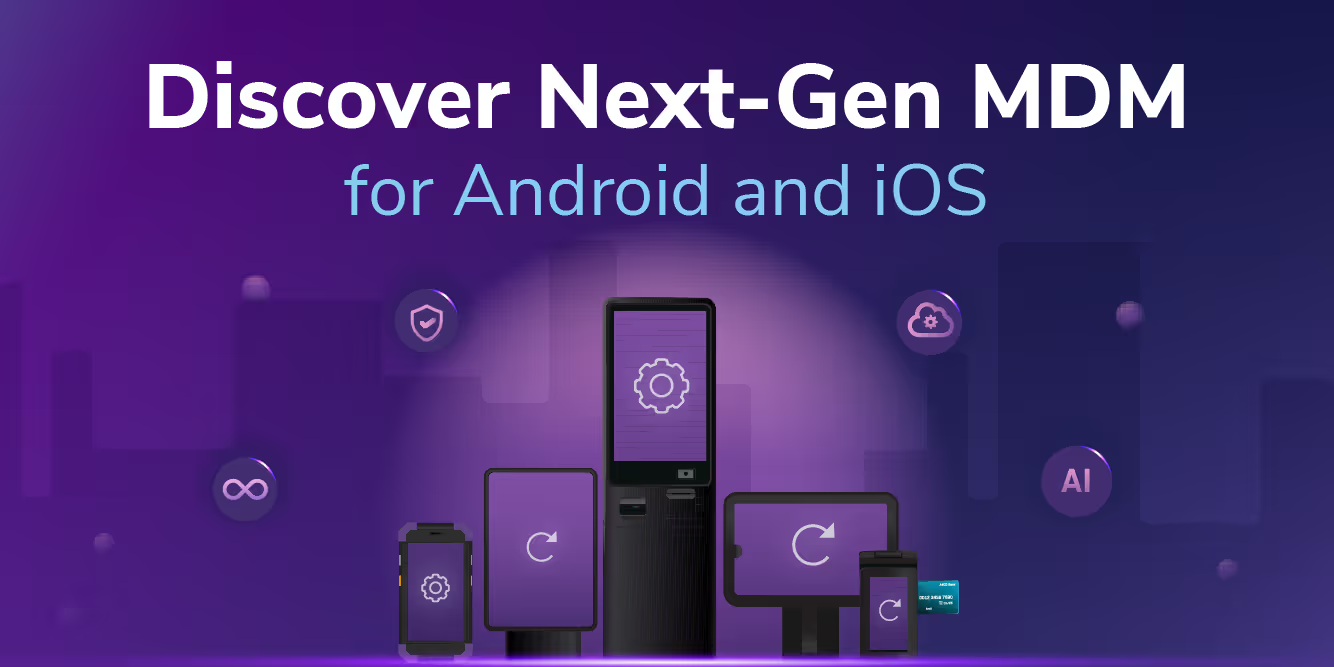