Edge devices are prolific in modern businesses — these business-critical pieces of hardware are mainstays for forward-thinking organizations to deliver next-level customer experiences. While they do critical work in retail stores, hospitals, vehicles, warehouses, restaurants, and more, they also collect a slew of valuable company data. If you’re not leveraging this data for fast (and I mean near real-time here) decision making, you’re leaving opportunities on the table.
What am I talking about? Crucial analytics data from edge devices — the smartphones, tablets, point of sale systems, digital kiosks, diagnostic equipment, and more that businesses rely on. Combining your edge devices with powerful AI tools that run on these devices, along with real-time analytics, enables next-level agility for your business!
In this article, I outline one possible solution for how you would employ AI with a widely distributed and multi-layered device and network topology. Of course, there are many strategies that could be employed. I’d love to hear from you if there are other ones that could benefit all of my readers!
Why the Edge?
First, let’s define what we mean by “the edge.” Edge devices are close to the source of data generation — a la on the edge of the network. Thinking about this hierarchically (and very simplistically), you have your company network at the very top. From there, you have each physical location with its own network. On that network, you have devices that perform various functions — kiosks, employee handhelds, barcode scanners, point of sale systems, MRI machines, X-ray machines, store-central compute, etc. Those are the furthest point from the company’s central network, but the closest to where data is generated — on the edge of the network.

So now you have these devices generating boatloads of valuable data. What do you do with that information? If you’re a forward-thinking company, you implement AI directly on those devices to process it in real time. That’s what edge AI is all about!
To make this a bit more concrete, let's consider a restaurant loyalty program. Wouldn’t you rather have the data about the customer processed right there, on the kiosk (or as close to the kiosk as possible) so the customer gets their service immediately? Contrast that to where the device generates information about the customer, ships that all the way to your cloud infrastructure, processes that centrally, and then ships information back to the device. Think of all the network latencies, large amounts of data (aka bandwidth), etc. involved. Not to mention the added cost!
Yes, the former is the much better option! You then upload only the important data to your central infrastructure for aggregate analysis and storage — much simpler!
Real-Time Analytics are the Key to an Agile Business
Okay, now you have devices gathering data and a way to generate real-time decisions based on that data. Where do you go from there? By leveraging that data for advanced analytics and faster short-term (i.e. not instant) decision making. This practice gathers and analyzes data in real-time, resulting in the ability to make adjustments quickly — and then pushes aggregate data to your central servers for higher-level processing. This is the beauty of analytics with edge AI — the real time decisions when you need them, along with aggregate data pushed to and processed on cloud services for better short and long term decision making (with automation)!
Here’s a possible scenario that could be employed for the best experience across the board:
- Make real-time decisions at the point of origin of data: Decide right at the kiosk how best to serve the customer — in real time
- Trend analysis in the store: Aggregate and analyze all the data that's generated in the store on a local server via an AI model. Identify trends, predict inventory needs, etc.
- Make smarter short and long term decisions: Push relevant aggregate data to your central infrastructure for more strategic analysis and decision-making
With this strategy, you not only service the customer immediately and make decisions as close to the source as possible but also save on network and bandwidth needs! Best of all worlds!
But wait, what does this have to do with AI?
Edge AI 🤝 Real-Time Analytics
By combining the power of AI running on the edge with analytics, you not only get access to real-time data, but an advanced, programmed AI to process that data and make actionable decisions on the spot. For example, AI could use analytics data to provide:
- Industrial Automation: Predictive maintenance, anomaly detection, quality control
- Healthcare: RPM, real-time device diagnostics in critical care situations
- Retail: Inventory optimization, in-store analytics
- Restaurant: Inventory management, restaurant analytics, market trends
In any of these scenarios (and many more), the device works for you on both ends — it’s there for quick and easy customer (or employee) interaction. Then, it uses the data to make fast decisions to optimize the device use, customer throughput, or even adjust for market trends as they happen. The options are pretty limitless here!
Challenges Associated with Edge AI and Analytics
Of course, that’s all pie in the sky if you can’t properly implement a solution. This is the hottest thing smoking on the edge scene right now and may seem relatively theoretical in the big picture. That’s particularly true, mainly because edge deployments continue to suffer from challenges outside of AI and analytics, like:
- Deploying models to the device: Whether you’re shipping models to a kiosk or to a hospital-central server, deploying models repeatedly and regularly continues to be a challenge
- Software deployment: Deploying any piece of software seamlessly to these devices
- Connectivity: Edge devices frequently suffer from poor network connections, complicating all data transfers in both directions
- Scalability: Whether you are dealing with a handful of devices in one store or have to manage hundreds of stores with thousands of devices, have a centralized and easy-to-use management tool that enables this
So, what’s the solution? Not to toot our own horn here, but it’s Esper. Deployments like this, where software delivery is paramount and scalability is critical, happen to be our specialty. If you’re interested in learning more about anything I’ve talked about here, chat with us!
FAQ
Keep Exploring
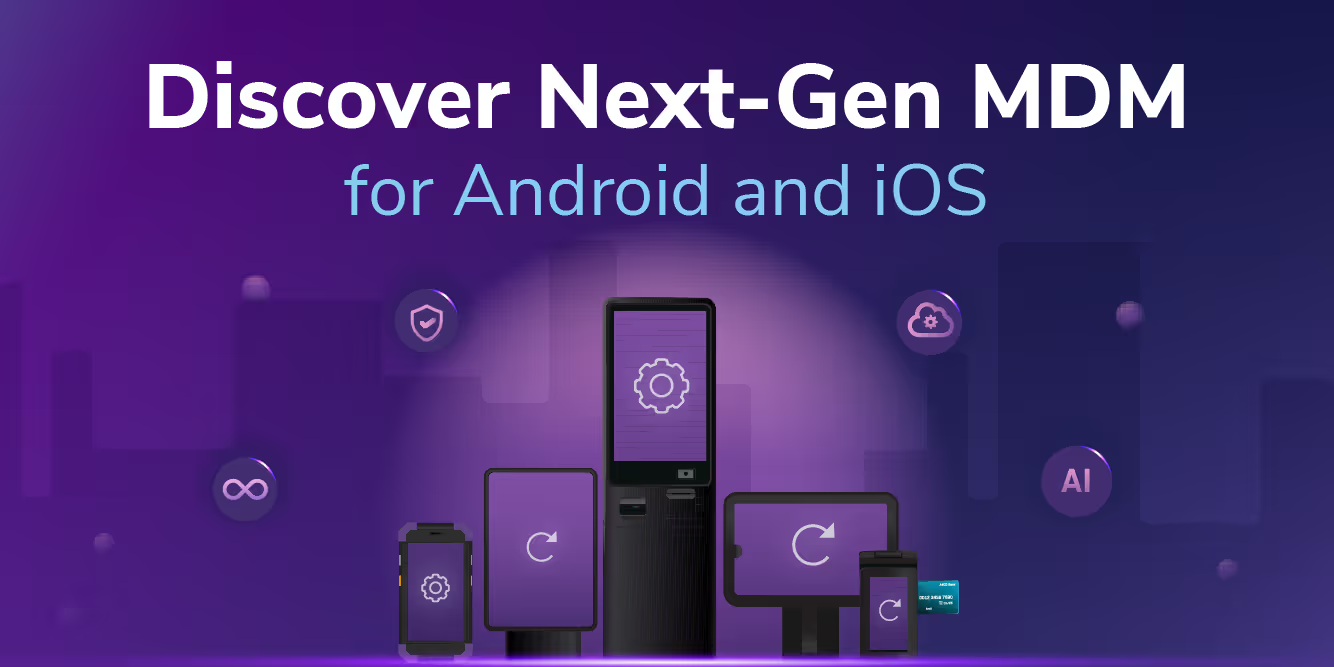